Fraud Detection and Prevention with Rule-Based Decision Engines
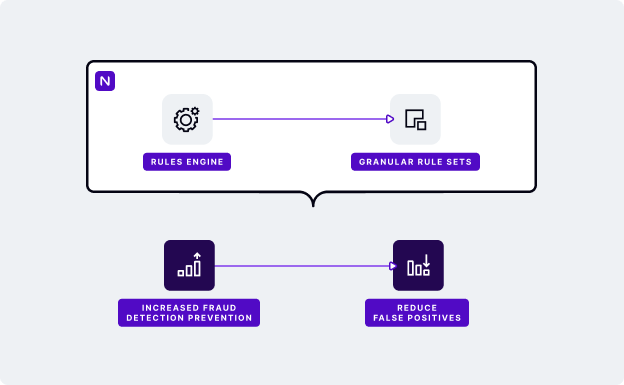
In the financial sector, rule-based decision engines play a crucial role in detecting and preventing fraud. These systems can process transactions in real-time, applying predefined rules to flag suspicious activities immediately.
Flexibility and Agility of Rule-Based Decision Engines
Rule-based decision engines allow for the rapid deployment of new rules and modifications. This capability is crucial in the fast-paced financial environment where threats can evolve rapidly. The ability to quickly implement changes helps financial institutions stay one step ahead of fraudsters, continuously adapting to new tactics and methods used in fraudulent activities.
Moreover, the ease of updating rules means that financial institutions can continuously refine and improve their fraud detection strategies. By analyzing the effectiveness of current rules and making data-driven adjustments, banks can enhance their fraud prevention measures, reducing both the incidence of fraud and the number of false positives that inconvenience legitimate customers.
Integrating Advanced Technologies for Robust Fraud Detection
Machine Learning for Pattern Recognition and Anomaly Detection
Machine learning (ML) models excel at recognizing patterns in vast datasets and identifying anomalies that may indicate fraudulent activity. Unlike traditional rule-based systems, which rely on predefined rules, ML algorithms can learn from historical data and adapt to new types of fraud. For example, ML can analyze transaction data in real-time to detect fraud, identifying irregularities that might be missed by static rules alone. This integration enhances the accuracy and efficiency of fraud detection.
Machine learning provides a complementary approach to rule-based systems, enabling more sophisticated analysis and the detection of subtle fraud patterns. By leveraging large volumes of historical transaction data, ML models can identify complex relationships and trends that might not be apparent through rule-based analysis alone. This ability to learn and adapt makes ML an invaluable tool in the fight against fraud.
AI for Dynamic and Predictive Analytics
AI technologies provide predictive analytics and real-time decision-making capabilities. AI systems can continuously learn and improve from new data, offering dynamic rule adjustments that keep pace with evolving fraud tactics. Techniques such as anomaly detection and risk scoring help financial institutions to preemptively identify and mitigate fraud risks, ensuring that they stay ahead of fraudsters.
AI enhances the capabilities of rule-based systems by introducing a layer of intelligence that can predict and respond to fraud in real-time. This predictive capability is essential for early detection and prevention, allowing financial institutions to address potential fraud before it results in significant losses. AI can also help prioritize investigations by scoring transactions based on their risk level, ensuring that resources are focused on the most suspicious activities.
Benefits of Combining Technologies
Combining rule-based decision engines with advanced technologies such as ML and AI offers several benefits:
Reduced False Positives: Ensures that legitimate transactions are not erroneously flagged, improving customer experience and operational efficiency.
Enhanced Fraud Detection Rates: Integrating AI and ML with rule-based engines allows for more accurate detection of fraudulent transactions. For example, PayPal’s use of these technologies has led to a substantial reduction in their fraud loss rate while handling increased transaction volumes.
Scalability and Flexibility: Advanced AI models can handle large-scale data processing and adapt to new fraud patterns without extensive manual intervention.
Real-World Applications
Several financial institutions have successfully implemented these integrated systems:
American Express: Uses generative AI to produce synthetic data that mimics fraudulent behavior, enhancing their fraud detection capabilities.
JP Morgan Chase: Employs machine learning and AI to analyze historical transaction data and identify patterns indicative of fraudulent behavior.
Citibank: Utilizes ML algorithms to uncover potential fraud rings by analyzing behavior patterns across multiple accounts.
Capital One: Uses deep learning models trained on vast historical data to detect forgeries, altered amounts, and counterfeit checks.
HSBC: Combines ML and rules engines to detect credit card fraud, providing transparency and identifying new fraud patterns.
Santander Bank: Utilizes trust and deny lists (rules engines) and ML models to detect new fraud patterns.
Deutsche Bank: Deploys an AI-powered fraud detection system that combines ML models with rules engines to monitor transactions in real-time.
Advanced Fraud Detection Techniques
To protect banking customers from fraud, financial institutions are investing heavily in advanced fraud detection techniques, such as:
Transaction Monitoring: Continuously monitoring transactions for signs of unauthorized activities.
Two-Factor Authentication (2FA): Adding an extra layer of security to prevent unauthorized access to customer accounts.
Anti Money Laundering (AML) Measures: Implementing robust AML protocols to detect and prevent money laundering activities.
Biometric Data: Utilizing biometric authentication methods to verify customer identities and prevent identity theft.
Fraud as a Service (FaaS): Keeping up with evolving fraud tactics offered as services on the dark web, ensuring proactive measures are in place.
Conclusion: Enhancing Security Through Technology
Three technology-based tools strengthen banking fraud protection and lower fraud risk: Artificial Intelligence, Machine Learning, and Rules Engines. These technologies provide comprehensive fraud detection and prevention capabilities, helping financial institutions stay ahead of fraudsters.
By integrating these technologies, banks can enhance their fraud detection strategies, reduce false positives, and improve operational efficiency. The combination of AI, ML, and rule-based decision engines offers a robust and scalable solution to the ever-evolving challenge of banking fraud, ensuring that financial institutions can protect their customers and their bottom line effectively.